Weekly Tech+Bio Highlights #39: Towards Human-Based Models
Also: AI News, NIH's Policy Shift & What Clinical AI Struggles With
Hi! This is BiopharmaTrend’s weekly newsletter, Where Tech Meets Bio, where we explore technologies, breakthroughs, and cutting-edge companies.
Note: we’ve recently launched a new report outlining a framework for what defines modern AI-driven drug discovery for 2025 and beyond.
If this newsletter is in your inbox, it’s because you subscribed, or someone thought you might enjoy it. In either case, you can subscribe directly by clicking this button:
Let’s get to this week’s topics!
🤖 AI x Bio
(AI applications in drug discovery, biotech, and healthcare)
🔹 The NIH launched a new initiative to reduce dependence on animal models by establishing an office tasked with advancing human-based research tools like organoids and computational models, aligning with the FDA’s parallel shift toward non-animal drug testing methods and broader regulatory reform.
🔹 FutureHouse, backed by Eric Schmidt, launched four AI agents—Crow, Falcon, Owl, and Phoenix—aimed at accelerating scientific discovery by assisting with literature analysis and experiment planning, though it has yet to achieve a breakthrough.
🔹 In a move to expand small-molecule discovery, Nurix Therapeutics unveiled a foundation model trained on billions of DNA-encoded library datapoints to predict binders for challenging protein targets, integrating ML with protein sequence inputs to support in silico screening and early-stage drug design.
🔹 Wiley and AWS launched an open-source AI agent that enables rapid full-text literature searches across Wiley’s content, aiming to shrink complex research tasks from days to minutes by integrating into AWS's multi-agent life sciences toolkit.—Brian Buntz, R&D World Online.
🔹 In early results from its clinical trial, Recursion reported that treatment with a MEK1/2 inhibitor developed using its AI-driven platform led to notable reductions in polyp burden among patients with Familial Adenomatous Polyposis.
🔹 With FDA clearance secured, Lantern Pharma is launching a Phase 1b/2 trial of its AI-designed drug for triple negative breast cancer.
🔹 Raidium published its foundation model for 3D tumor segmentation in npj Precision Oncology, presenting a promptable, CT-based tool that lets radiologists interactively define and refine lesion boundaries.
🔹 Researchers from Ontario Tech, Brock, and Laurier universities conducted an analysis of bias in histopathology AI models, showing that cancer type predictions can be confounded by data center-specific signatures embedded in the TCGA dataset due to staining variations, patch processing, and imbalanced sampling.
🔹 AI systems failed to detect 66% of life-threatening hospital injuries, according to a study published in Nature Communications Medicine and reported by Axios' Maya Goldman. The research found that leading machine learning models used in hospitals—trained solely on past patient data—missed most critical cases when tested under variable conditions, underscoring the limitations of purely data-driven approaches in real-world care. “Purely data-driven training alone is not sufficient,” said study co-author Danfeng Yao of Virginia Tech.
🔹 Iambic unveils Enchant v2, a next-gen multimodal AI model that predicts key clinical, safety, and pharmacological properties for drug candidates with limited data, reportedly outperforming traditional in vitro methods in some tasks.
🔹 Chan Zuckerberg Initiative researchers publish TranscriptFormer, a generative model trained on 112 million single cells from 12 species, designed to organize gene expression data without labels and generalize across evolutionary distances, enabling tasks like cell type prediction and virtual probing of transcription factor relationships.
🔹 Shanghai Jiao Tong researchers publish a landmark survey on AI agent protocols, introducing a two-dimensional taxonomy for classifying LLM agent communication standards and evaluating key protocols like MCP, A2A, ANP, and Agora on efficiency, security, scalability, and interoperability—proposing that standardized, evolvable protocols will power future collective intelligence infrastructures.
🚜 Market Movers
(News from established pharma and tech giants)
🔹 Eli Lilly partners with Creyon Bio in a deal worth up to $1 billion ($13M upfront) to develop AI-designed oligonucleotide therapeutics, gaining exclusive rights to candidates from Creyon’s machine learning platform that engineers RNA and DNA molecules to modulate gene expression.
🔹 Merck breaks ground on a $1B biologics center in Wilmington, DE, aiming to support production of next-gen biologics including KEYTRUDA, with the site expected to create 500+ full-time jobs and 4,000 construction roles, and serve as a U.S. hub for Merck’s expanding biologics portfolio.
🔹 Roche’s AI-powered Ventana TROP2 test wins FDA breakthrough status as the first AI companion diagnostic for lung cancer, guiding use of ADC therapy Datroway.
🔹 Vertex ends development of AAV-based gene therapies amid broader industry retreat from the viral vector, while reaffirming its commitment to cell and genetic therapies through assets like Casgevy and zimislecel for sickle cell, thalassemia, and Type 1 diabetes.
💰 Money Flows
(Funding rounds, IPOs, and M&A for startups and smaller companies)
🔹 Basil Systems secures $11.5M led by Golden Ventures to expand its AI-powered SaaS platform for regulatory and product lifecycle intelligence in pharma and MedTech.
🔹 Pathos AI, a Chicago biotech led by former Tempus executives, has received funding from AstraZeneca and is planning to raise up to $400M.
🔹 Novartis to acquire Regulus Therapeutics to advance farabursen, a first-in-class microRNA-targeting therapy for ADPKD, aiming to address a major unmet need in renal disease with enhanced safety and efficacy over current standards.
🔹 HistoSonics, developer of the Edison histotripsy system that non-invasively destroys liver tumors using focused ultrasound, is reportedly in early acquisition talks with Medtronic, GE HealthCare, and J&J after strong clinical data and FDA clearance, potentially valuing the company at over $2.5B.
🔹 Chipiron raises $17M to develop a new generation of compact, affordable MRI systems designed for frontline and underserved healthcare settings, aiming to improve accessibility and speed of care with deployable, patient-centric imaging technology.
⚙️ Other Tech
(Innovations across quantum computing, BCIs, gene editing, and more)
🔹 Aiming to limit off-target toxicity in cancer therapy, researchers developed a pH-sensitive DNA origami device that displays cytotoxic ligands only under acidic conditions typical of tumor microenvironments, enabling selective apoptosis induction in cancer cells while remaining inert in healthy tissue, with early in vivo tests showing up to 70% tumor suppression.
🔹 The AllTheBacteria project has made over 2 million bacterial and archaeal genomes publicly available, complete with gene annotations and antibiotic resistance data, allowing scientists to search and compare genetic information quickly using free tools online or through AWS.
🏛️ Bioeconomy & Society
(News on centers, regulatory updates, and broader biotech ecosystem developments)
🔹 No clear winner—in a first-of-its-kind adversarial study, Cogitate Consortium, spanning institutions like Caltech and the Allen Institute, spent seven years testing rival theories of consciousness through a preregistered, multi-method brain imaging experiment, aiming to reduce bias and set a new standard for resolving foundational scientific disputes.
🔹 Aviva Capital Partners and developer Socius unveiled a £1B plan to build the “London Cancer Hub” in Sutton, aiming to create 3,000 high-skill jobs and expand the UK’s cancer R&D capabilities with 1M sq. ft. of lab and manufacturing space near the Golden Triangle.
🔹 U.S. biotech firms are increasingly shifting clinical trials abroad amid growing concerns over regulatory uncertainty and leadership changes at the FDA, as exemplified by Siren Bio’s CEO considering international alternatives following Peter Marks’ resignation.
🔹 HHS launches a $500M “Generation Gold Standard” initiative to develop universal vaccines using chemically inactivated whole-virus platforms, aiming for broad protection against pandemic-prone viruses like influenza and coronaviruses.
🔹 Verily secures a $14.7M grant from the Michael J. Fox Foundation to expand the Personalized Parkinson’s Project, integrating wearable data, molecular profiling, and imaging to build one of the most detailed real-world datasets on Parkinson’s disease for biomarker discovery and therapeutic development.
🚀 A New Kid on the Block
(Emerging startups with a focus on technology)
🔹 Stately Bio emerges from stealth with $12M seed funding led by AIX Ventures, applying computer vision and AI to cellular biology—founded by ex-Calico machine learning head Frank Li to explore image-based insights.
🔹 Twist Bioscience has spun out Atlas Data Storage as a separate company with $155 million in backing to commercialize its DNA-based data storage technology.
This newsletter reaches over 8.5K industry professionals from leading organizations across the globe. Interested in sponsoring?
Contact us at info@biopharmatrend.com
NIH Shifts Focus to Organoids and Digital Models
The NIH has opened a new Office of Research Innovation, Validation, and Application (ORIVA) to help scale human-based research technologies across its biomedical portfolio. This includes increased funding and infrastructure for organoids, tissue chips, computational modeling, and real-world data approaches.
The initiative also includes expanded training programs aimed at increasing the workforce proficient in non-animal methods.
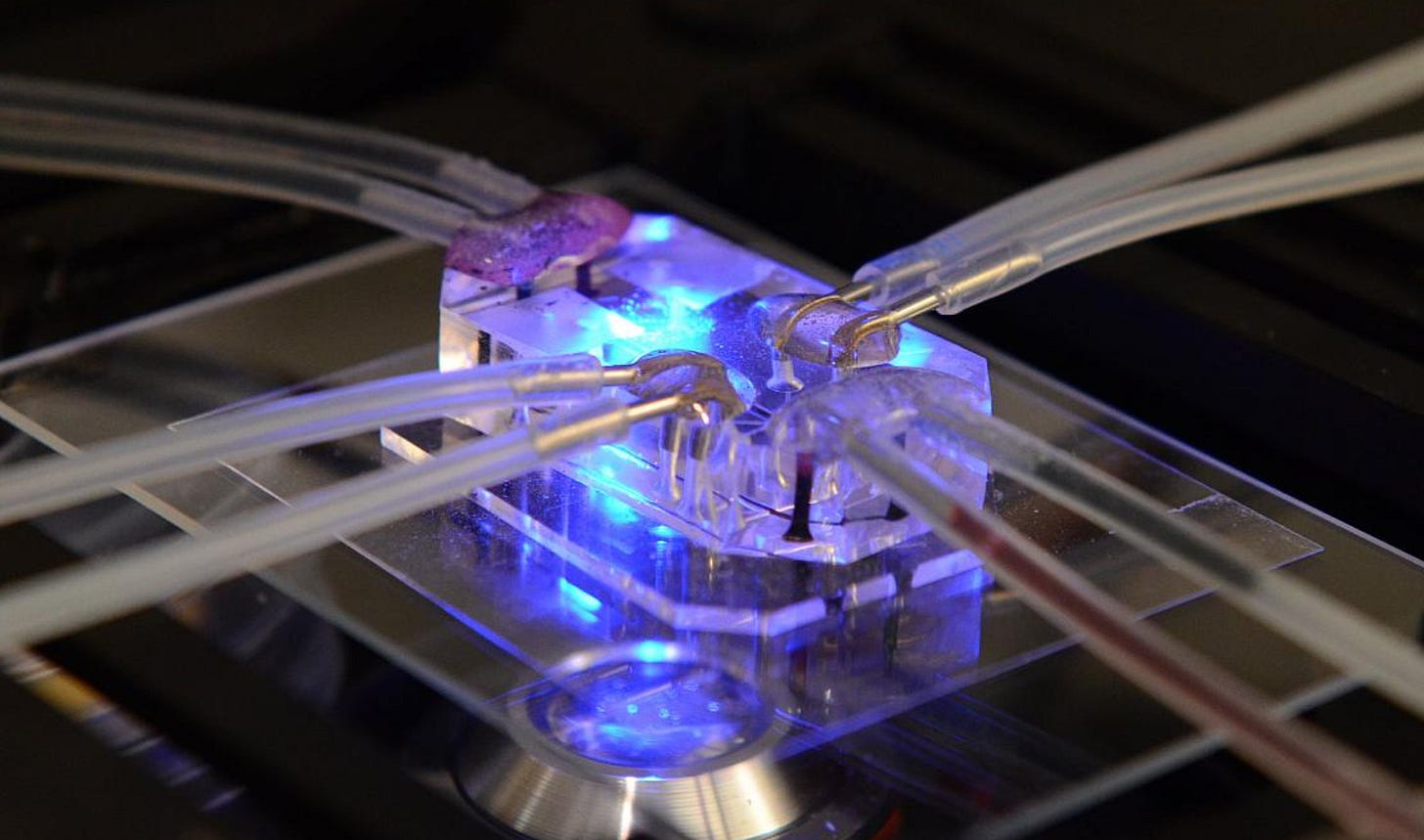
The news follows the FDA’s April 2025 roadmap to scale back animal testing requirements. That policy outlines support for New Approach Methods (NAMs)—including organ-on-chip systems, AI-driven toxicity prediction, and human-derived organoids—as acceptable evidence in investigational new drug (IND) applications. Submissions using NAMs may qualify for faster reviews if data quality is deemed robust.
NIH will also revise its grant review process to prioritize human relevance and translational potential, and will begin publishing annual reports on its use of animal models.
These reforms will be coordinated with other federal agencies—including the FDA and the Department of Veterans Affairs—through the Interagency Coordinating Committee on the Validation of Alternative Methods (ICCVAM).
A pilot program focused on monoclonal antibody development is being prepared and is expected to guide further policy updates.
What Clinical AI Struggles With
An in-depth evaluation recently published in Nature Communications Medicine reveals that many clinical ML models fail to detect or respond to deteriorating patient conditions, including abnormal vital signs and severe injury indicators. The study raises concerns about their readiness for deployment in critical care settings and suggests future models must incorporate explicit medical knowledge.
Most AI models tested failed to spot critical patient deterioration: Many machine learning models couldn't recognize when a patient's condition became dangerously worse, even with very abnormal vital signs.
66% of critical injuries went undetected: In synthetic test cases for predicting in-hospital mortality, the models missed two-thirds of the serious conditions that should have triggered alerts.
Models often ignored dangerous vital sign changes: For example, models didn’t respond adequately to slow breathing (bradypnea), low oxygen levels (hypoxemia), or high body temperatures (hyperthermia).
Even advanced models like LSTM and Transformers missed critical cases: While transformers performed slightly better than traditional models, they still failed to detect several high-risk health states.
Cancer prediction models had similar blind spots: Models predicting 5-year breast and lung cancer survival were often unresponsive to worsening disease indicators like large tumors or extensive lymph node involvement.
Adding more training data from rare cases didn’t help much: Techniques like oversampling rare cases or reweighting classes only slightly improved model performance and sometimes made it worse.
Simple models outperformed deep learning in some cases: For categorical data like Glasgow Coma Scale scores, a basic logistic regression model gave more consistent predictions than complex neural networks.
Neural networks often had ‘dead zones’: Internal neuron activity showed that many model components didn’t react to critical changes in inputs, indicating they didn’t learn to recognize certain medical emergencies.
Most test data used in model training lacks severe cases: This results in models being poorly prepared for real-life situations that are outside the range of the original data.
Future models may need medical knowledge built-in: The study suggests that AI models should incorporate clinical guidelines or medical reasoning, not just rely on historical patient data.
These findings underscore a key limitation in many clinical AI systems: while they may perform well on average-case benchmarks, they often fail in high-risk edge cases where accurate prediction matters most. The authors suggest that without structural changes—such as incorporating clinical priors or explicitly testing for extreme scenarios—these models may remain unreliable for critical decision support.
Read also:
13 Foundation Models: Startups, Industry Updates and the Nobel Prize