The Infrastructure Layer: Platforms Powering Human-Relevant Drug Development
Infrastructure for large-scale biomedical data—from multi-omics to EHRs—is converging with a growing policy and business case for data-native drug development.
In recent weeks, several announcements captured where data-driven biomedicine is heading. Google released a whole-brain zebrafish benchmark capturing 2-hour activity from over 70,000 neurons; Tempus, AstraZeneca, and Pathos committed $200 million to train a foundation model on multimodal cancer data drawn from Tempus’ clinical-genomic archive; Immunai and the Parker Institute are assembling a 3,700-sample single-cell dataset from 1,000 immunotherapy patients, paired with multi-omic and clinical data to map response and resistance patterns.
In this article: Clinical Data & Real-World Evidence Platforms — Biobank & Biological Data Platforms — Electronic Health Record Data Enrichment & Integration — Genomic & Multi-Omics Big Data Companies — Clinical Trial Data & Decentralized Trial Platforms — AI-Driven Drug Discovery Platforms — Wearable & Patient-Generated Health Data Platforms
In parallel, over 100 researchers working under the MICrONS program released a map of one cubic millimeter of mouse brain tissue. The team, led by the Allen Institute, Princeton, and Baylor College of Medicine, reconstructed 200,000 cells and 523 million synapses using 28,000 brain slices. The resulting dataset is 1.6 petabytes of high-resolution imaging paired with in vivo activity recordings.
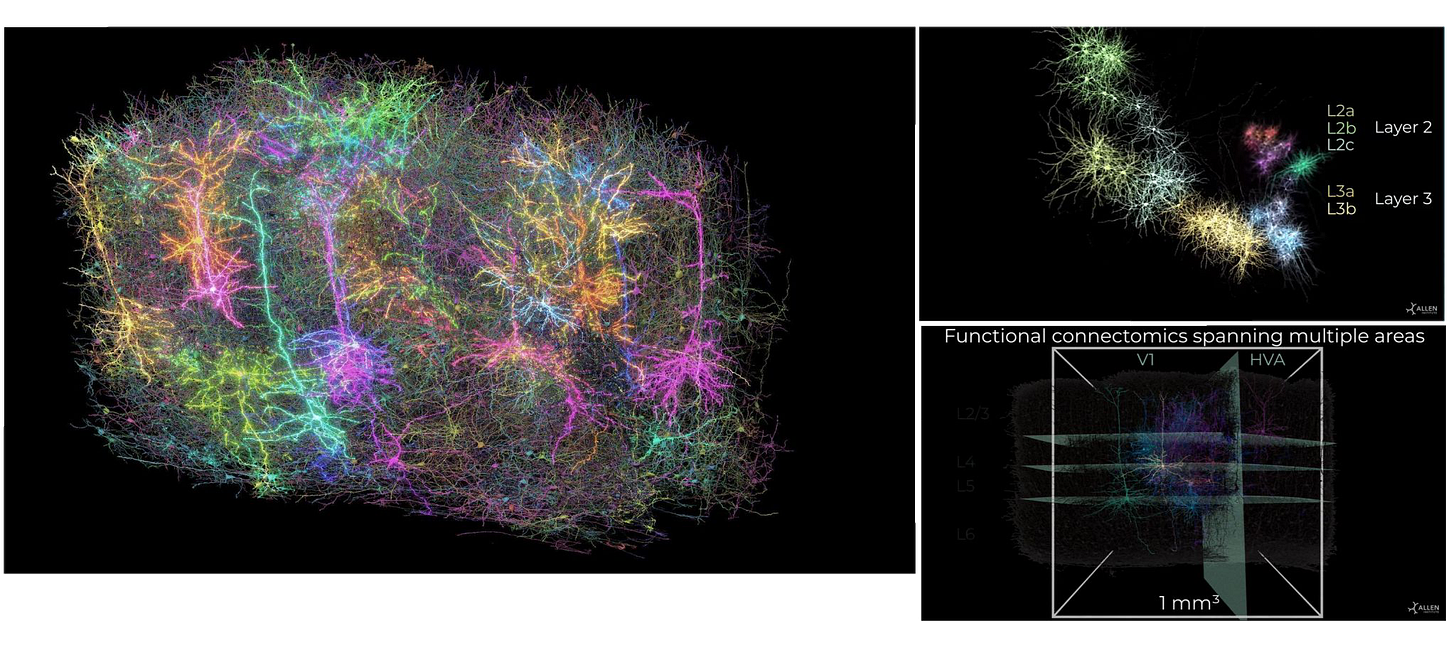
It’s less than 1% of a mouse brain. But it already revealed new circuit motifs involving inhibitory neuron types like chandelier and Martinotti cells. The dataset is open access and intended as a scaffold for future precision neurotherapeutics.
Looking back to 1965, Margaret Dayhoff compiled just 65 protein sequences in her Atlas of Protein Sequence and Structure. The entire dataset fits into a few kilobytes—small enough to store on a floppy disk or email as a modern PDF. Today, a single biomedical project can generate petabytes. That’s a billion-fold increase in volume.
This kind of output, unthinkable two decades ago, is now routine in many areas of biomedicine. GenBank stores over 250 billion nucleotide bases. UK Biobank holds more than 30 petabytes across genomic, imaging, and health data. Tempus AI processes 300 petabytes across multimodal clinical and molecular datasets. AlphaFold’s 200 million protein predictions add another 23 terabytes of modeled biology.
What made this scale-up possible? Faster compute, cheaper storage and better compression. And a growing ecosystem of tools to ingest, clean, and analyze multi-source biomedical data in real time.
In a major policy shift, the FDA had just expanded the use of New Approach Methodologies (NAMs) in Investigational New Drug (IND) submissions, starting with monoclonal antibodies and other therapeutics. The update allows developers to replace certain animal studies with validated alternatives, including in silico models, human cell assays, and organoid systems. This move builds on the FDA Modernization Act 2.0, which in 2022 removed the federal requirement for animal testing in some cases. The regulatory framework is now shifting toward more modern, mechanistic, and human-relevant tools in early-stage drug development.
According to Enke Bashllari, founder and managing director at Arkitekt Ventures, these shifts open up significant opportunities for startups focused on AI-driven prediction, modeling, and simulation in drug development.